Minimal spectral HQI for automatic detection: Are we introducing false positives by relying too much on automation?
- Oskar Hagelskjær
- Nov 25, 2024
- 3 min read
The field of microplastic science has long suffered from a lack of methodology harmonization, however, this is slowly starting to change. Major standardization bodies such as the ISO and the EU, are now publishing preliminary workflow drafts. See ISO/DIS 16094-2 and Article 13(6) of EU Directive 2020/2184.
While this is good news for the standardization of microplastic research, it sparks the discussion on what are important analytical aspects. It is important that the selected requirements are analytically robust. Most of us can agree on the importance of blanks and recovery experiments, but when we dig into the details, we tend to base our interpretation of the 'correct approach' on our own experience with laboratory work, data treatment and so on - and these might all vary based on our types of equipment, workflows, sample types, etc.
Devil's in the details
One such is example is acceptable HQI (hit quality index) thresholds of spectral matches. During my Ph.D., it appeared that in our team, we all used slightly different spectral libraries, and the way in which we sorted and intrepreted the results were different too! Imagine then a research team in another country, with different intruments and software. It would be miracolous if we were to repeat the same results in a similar sample - but this is a whole nother discussion - If you're interested in learning more about interlaboratory comparison, I recommend this article by De Frond et al. (2022). The results are eye-opening.
Back to HQI
HQI is the end-of-the-line, i.e. the determining factor of the identification of a microplastic particle. Therefore, it is obviously very important that we can agree on how HQI is interpreted. The ISO/DIS 16094-2 suggets the following approach to automated identification:
"The laboratory will have to demonstrate a similarity (difference of less than 30 % of false-positive or false-negative) of the results obtained with the use of the automatic recognition algorithm compared to results obtained using visual processing by a qualified operator". - ISO/DIS 16094-2.
As far as I can interpret, this means that a common HQI is determined for all polymers as a whole, and not for the individual polymer types (Fig. 1). Do please correct me in the comments, if I'm wrong!

Fig. 1 - Excerpt of the ISO/DIS 16094-2 demonstrating the suggeted apprach to spectral matching and verification.
However, even if this is not the case, It's my experience that not only do you have to set individual HQI thresholds for indiviudal polymer types - because some are more easily misidentified - but you should also adapt HQI threshold based on different sample matrices. This is important because organic compounds in the specific matrix, may closely ressemble one or more polymer types.
Here's an example: polyamides!
During my Ph.D. I worked a lot on microplastic concentrations and polymer type disitributions in peat, specifically Sphagnum mosses. Here I stumbled upon a compound in the peat (which persisted even after chemical treatment), which would match very well with polyamide 6 and 6,6 with up to 98% HQI - although it clearly was not! (Fig. 2).
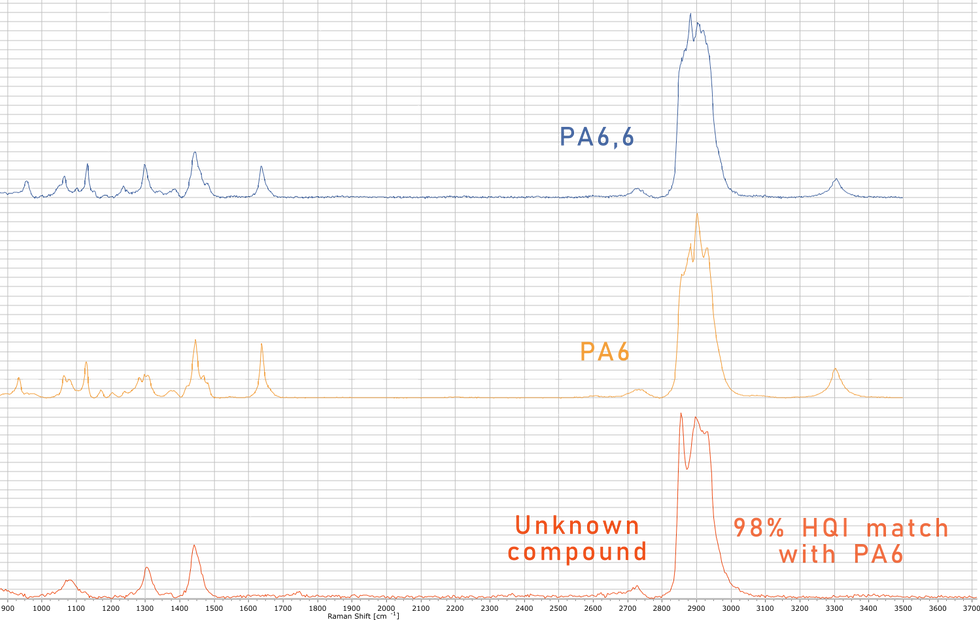
Fig. 2 - Comparison of Raman spectra of (from top to bottom) PA6, PA6,6 and an unknown compound found the Sphagnum moss, which matched with PA6 or PA6,6.
One might argue that false positive spectra should be added to the spectral library, to avoid these types of misidentifications (if we rely solely on automation). However, realistically this is unfeasible for two reasons.
1) It is simply unfeasible to gather chemical information of all existing compounds.
2) And two, even if you were able to gather all this data - cross examining the thousands or millions of spectra would require a lifetime. Yes, and that is by automated comparison analysis. Currently, cross examining a spectral library of 100 polymer spectra with ~20,000 acquired samples spectra, takes 30-40 minutes. If you add 100 spectra more to your library, the analysis time is effectively doubled.
For this reason, I urge to always Inspect the spectral matches yourself - If you have oly a handful of matches check them all individualy - if you have hundreds of matches (like PA6 in peat), check out some of the best matches and decide at what HQI value the matches become unreliable, and set a cutoff for this particlular polymer type.
In conclusion
HQI alone is insufficient to determine whether a spectral match is true or not. Instead HQI is a tool that helps you sort through your data. It is very likely though, that in the future, artificial intelligence and deep learning structures will be able to more intelligently segment and compare spectra.
What's your opinion and experience?
Comments